μ λ 1.2.1 λ²μ μ κ°λ°μ©μΌλ‘ μ¬μ©νκ³ μμ΅λλ€. νμ΄μ¬μΌλ‘ μ€μΉκ° μ½κΈ° λλ¬Έμ λλ€. λν c_apiλ₯Ό μ¬μ©νμ¬ μμΈ‘μ μν κ°λ¨ν c μ½λλ₯Ό μμ±νκ³ μμ΅λλ€. v1.2.1 libxgboost.soμ μ°κ²°λ κ²½μ° pythonκ³Ό c κ°μ μμΈ‘ μ°¨μ΄λ μ νν 0μ λλ€. κ·Έλ¬λ λ§μ€ν° λΈλμΉμμ libxgboost.soλ‘ μ°κ²°νλ©΄(2020λ 11μ 5μΌ f3a425398 컀λ°) μ°¨μ΄κ° μμ΅λλ€.
μ μ libλ₯Ό ꡬμΆνκ³ μΆκΈ° λλ¬Έμ master λΈλμΉλ₯Ό μ¬μ©νμ¬ μ€μ μμ€ν μ c μ½λλ₯Ό λ°°ν¬νκ³ μΆμ΅λλ€. μ΄μ v1.2.1κ³Ό master λΈλμΉμ μμΈ‘ μ°¨μ΄κ° μ λ₯Ό λ°©ν΄ν©λλ€.
κ°μ¬ ν΄μ.
λ²κ·Έλ₯Ό μ¬νν μ μλλ‘ μμ νλ‘κ·Έλ¨μ κ²μν μ μμ΅λκΉ?
λν Python ν¨ν€μ§μ λ§μ€ν° λ²μ κ³Ό C APIμ μμΈ‘μ΄ λ€λ₯Έκ°μ?
CPU μμΈ‘μμμ μνλ μΌλΆ μ΅μ νκ° μμΌλ©° λ€λ₯Έ λΆλ μμμ μ€λ₯λ‘ μΈν΄ λ€λ₯Έ κ²°κ³Όλ₯Ό μμ±ν μ μμ΅λλ€. κ·Έλ¬λ μ, μ¬ν κ°λ₯ν μκ° μμ΅λκΉ?
λν Python ν¨ν€μ§μ λ§μ€ν° λ²μ κ³Ό C APIμ μμΈ‘μ΄ λ€λ₯Έκ°μ?
python ν¨ν€μ§μ v1.2.1κ³Ό C APIμ λ§μ€ν° λΈλμΉλ₯Ό λΉκ΅νκ³ μμ΅λλ€.
@7starsea λ§μ€ν° λΈλμΉμ Pythonκ³Ό C APIμ μΆλ ₯λ λΉκ΅ν μ μμ΅λκΉ? λ¬Έμ λ μ ν리μΌμ΄μ μμ C API ν¨μκ° μ¬μ©λλ λ°©μμΌ μ μμ΅λλ€.
@7starsea λ§μ€ν° λΈλμΉμ Pythonκ³Ό C APIμ μΆλ ₯λ λΉκ΅ν μ μμ΅λκΉ? λ¬Έμ λ μ ν리μΌμ΄μ μμ C API ν¨μκ° μ¬μ©λλ λ°©μμΌ μ μμ΅λλ€.
λλ v1.2.1μ pythonκ³Ό c apiλ₯Ό λΉκ΅νκ³ μμΈ‘μ μ νν λμΌν©λλ€.
@7starsea μκ² μ΅λλ€. λμΌν λͺ¨λΈμμ μμΈ‘νλ Python λ° C νλ‘κ·Έλ¨μ λͺ¨λ κ²μνλ©΄ λ¬Έμ λ₯Ό μΆκ°λ‘ ν΄κ²°ν μ μμ΅λλ€.
@hcho3 μ ν μ€νΈ μ½λμ λλ€.
@7starsea λ°©κΈ κ·νμ μλ₯Ό μλνκ³ λ€μκ³Ό κ°μ κ²°κ³Όλ₯Ό μ»μμ΅λλ€.
difference: [0. 0. 0. 0.] 0.0 0.0
XGBoostμ μ΅μ 컀λ°(debeae2509d90ec1d3402a3a185fba7a25113ff1)μ μ¬μ©νμ΅λλ€.
@7starsea λ°©κΈ κ·νμ μλ₯Ό μλνκ³ λ€μκ³Ό κ°μ κ²°κ³Όλ₯Ό μ»μμ΅λλ€.
difference: [0. 0. 0. 0.] 0.0 0.0
XGBoost( debeae2 )μ μ΅μ 컀λ°μ μ¬μ©νμ΅λλ€.
ν₯λ―Έλ‘μ΅λλ€. νμ΄μ¬ λ²μ μ΄ v1.2.1μ λκΉ?
λλ μ¬μ ν python-version 1.2.1κ³Ό XGBoost( debeae2 )μ μ°κ²°λ c api μ¬μ΄μ μ½κ°μ μ°¨μ΄κ° μμ΅λλ€.
@7starsea μλμ, μ΅μ μμ€(commit debeae2509d90ec1d3402a3a185fba7a25113ff1)μμ XGBoostλ₯Ό μ»΄νμΌ νμΌλ―λ‘ v1.2.1λ³΄λ€ μ΅μ μ
λλ€. λ΄ XGBoost Python ν¨ν€μ§λ xgboost.__version__
νλμ λν΄ 1.3.0-SNAPSHOT
λ₯Ό μΈμν©λλ€.
@hcho3 XGBoost κ° λ€λ₯Έ λ²μ (μ΅μν μ°μ λ²μ ) κ°μ μΌκ΄λ μμΈ‘μ μ μ§ν΄μΌ νλμ§ κΆκΈν©λλ€.
λν v1.3.0μ μΆμλ₯Ό κΈ°λνκ³ μμ΅λλ€.
(μ§κΈ λ§μ€ν° λΈλμΉλ₯Ό μ¬μ©νμ¬ λͺ¨λΈμ νλ ¨μμΌμΌ ν κ² κ°μ΅λλ€)
μκ° λ΄ μ€μ κ³ λ§μ.
@7starsea μ΄μ λ²μ μμ μ μ₯λ λͺ¨λΈμ
κ·νμ μ€ν¬λ¦½νΈλ₯Ό μ¬μ©νμ¬ λ¬Έμ λ₯Ό μ¬νν μ μμ΅λλ€. Docker μ΄λ―Έμ§λ VM μ΄λ―Έμ§λ₯Ό λΉλνκ³ κ³΅μ ν΄ μ£Όμκ² μ΅λκΉ?
@7starsea μ°Έκ³ λ‘, λ€μκ³Ό κ°μ΄ μμ€μμ XGBoost 1.2.1 λΉλλ μλνμ΅λλ€.
git clone --recursive https://github.com/dmlc/xgboost -b release_1.2.0 xgb_source
cd xgb_source
mkdir build
cd build
cmake ..
make
cd ../python-package
python setup.py install
κ²°κ³Όκ° λ€μ difference: [0. 0. 0. 0.] 0.0 0.0
μ°¨μ΄μ μ νμΈνλ €λ©΄ λ κ°μ§ λ²μ μ XGBoost, Pythonμ© v1.2.1 λ°
dtest = xgb.DMatrix(rx, missing=0.0)
y1 = m2.predict(dtest) # # internally using libxgboost.so v1.2.1
κ·Έλ¦¬κ³ νλλ cppμ©
m1 = XgbShannonPredictor(fname)
y2 = m1.predict(rx2) # # internally using libxgboost.so from the master branch (debeae2)
λλ (λμκ² μλ‘μ΄) λ컀 μ΄λ―Έμ§λ₯Ό λ§λ€λ €κ³ λ Έλ ₯ν κ²μ΄λ€.
ν λ² λ³Όκ²μ.
λλ (λμκ² μλ‘μ΄) λ컀 μ΄λ―Έμ§λ₯Ό λ§λ€λ €κ³ λ Έλ ₯ν κ²μ΄λ€.
νμνμ§ μμ΅λλ€.
μ€μ λ‘ λ¬Έμ λ₯Ό μ¬ννλ λ° μ±κ³΅νμ΅λλ€. XGBoostμ dev λ²μ μ XGBoost 1.2.0κ³Ό λ€λ₯Έ μμΈ‘μ μμ±νλ κ²μΌλ‘ λνλ¬μ΅λλ€. κ·Έλ¦¬κ³ λ¬Έμ λ μ¬ννκΈ° μ½μ΅λλ€. C APIλ₯Ό μ¬μ©ν νμκ° μμ΅λλ€.
μ¬ν κ°λ₯ν μ(νΈμ§: λλ€ μλ μ€μ ):
import numpy as np
import xgboost as xgb
rng = np.random.default_rng(seed=2020)
rx = rng.standard_normal(size=(100, 127 + 7 + 1))
rx = rx.astype(np.float32, order='C')
m2 = xgb.Booster({'nthread': '4'}) # init model
m2.load_model('xgb.model.bin') # load data
dtest = xgb.DMatrix(rx, missing=0.0)
y1 = m2.predict(dtest)
print(xgb.__version__)
print(y1)
1.2.0μ μΆλ ₯:
1.2.0
[ 0.00698659 -0.00211251 0.00180039 -0.00016004 0.00526169 0.00801963
0.00016755 0.00226218 0.00276762 0.00408182 0.00303206 0.00291929
0.01101092 0.0068329 0.00145864 0.00326979 0.00572816 0.01019934
0.00074345 0.00784767 0.00173795 -0.00219297 0.0060181 0.00606489
0.00447372 0.00103396 0.00932363 0.00230178 0.00389203 0.00151157
0.0034163 0.00821933 0.006686 0.00630778 0.00331488 0.00775066
0.00443819 0.01030204 0.00924486 0.00645933 0.00777653 0.00231206
0.00457835 0.00390425 0.00947028 0.00410065 0.00220913 0.00292507
0.00637993 0.00796807 0.00140873 0.00887537 0.00496858 0.01049942
0.00908098 0.00332722 0.00799242 0.00228494 0.00463879 0.00213429
0.00729388 0.01049232 0.00790522 0.01269361 -0.00425893 0.00256333
0.00859573 0.00472835 0.00077197 0.00191873 0.01546788 0.0014475
0.00888193 0.00648022 0.00115797 0.00351191 0.00580138 0.00614035
0.00632426 0.00408354 0.00346044 -0.00034332 0.00599384 0.00302595
0.00657633 0.01086903 0.00625807 0.00096565 0.00061804 0.00038511
0.00523874 0.00633043 0.00379965 0.00302553 -0.00123322 0.00153473
0.00725579 0.00836438 0.01295918 0.00737873]
λ©λͺ¨. XGBoost 1.0.0 λ° 1.1.0μΌλ‘ μ€ν¬λ¦½νΈλ₯Ό μ€ννλ©΄ 1.2.0κ³Ό λμΌν μΆλ ₯μ΄ μμ±λ©λλ€.
κ°λ° λ²μ μ μΆλ ₯(c5645180a6afb9d3d771165e681985fe3522adf6)
1.3.0-SNAPSHOT
[ 0.00698666 -0.00211278 0.00180034 -0.00016027 0.00526194 0.00801962
0.00016758 0.00226211 0.00276773 0.00408198 0.00303223 0.00291933
0.01101091 0.00683288 0.00145871 0.00326988 0.00572827 0.01019943
0.00074329 0.00784767 0.00173803 -0.00219286 0.00601804 0.00606472
0.00447388 0.00103391 0.00932358 0.00230171 0.003892 0.00151177
0.00341637 0.00821943 0.00668607 0.00630774 0.00331502 0.00775074
0.0044381 0.01030211 0.00924495 0.00645958 0.00777672 0.00231205
0.00457842 0.00390424 0.00947046 0.00410091 0.0022092 0.00292498
0.00638005 0.00796804 0.00140869 0.00887531 0.00496863 0.01049942
0.00908096 0.00332738 0.00799218 0.00228496 0.004639 0.00213413
0.00729368 0.01049243 0.00790528 0.01269368 -0.00425872 0.00256319
0.00859569 0.00472848 0.0007721 0.00191874 0.01546813 0.00144742
0.00888212 0.00648021 0.00115819 0.00351191 0.00580168 0.00614044
0.00632418 0.0040833 0.00346038 -0.00034315 0.00599405 0.00302578
0.0065765 0.01086897 0.00625799 0.00096572 0.00061766 0.00038494
0.00523901 0.00633054 0.00379964 0.00302567 -0.00123339 0.00153471
0.00725584 0.00836433 0.01295913 0.00737863]
@hcho3 μ΄ν΄
μ κΉλ§μ, μ μ¬νμ 무μμ μλλ₯Ό μ€μ νλ κ²μ μμμ΅λλ€. λ°λ³΄ λ.
κ³ μ λλ€ μλλ‘ μ¬νμ μ λ°μ΄νΈνμ΅λλ€. λ²κ·Έκ° μ¬μ ν μ§μλ©λλ€. μ λ°μ΄νΈλ μ¬νμ XGBoost 1.0.0 λ° 1.1.0μΌλ‘ μ€νν΄ λ³΄μλλ° μμΈ‘μ΄ XGBoost 1.2.0μ μμΈ‘κ³Ό μΌμΉν©λλ€.
κ°λ¨ν λ§ν΄μ:
Prediction from 1.0.0
== Prediction from 1.1.0
== Prediction from 1.2.0
!= Prediction from latest master
@trivialfis μ, λμμ μ£Όμλ©΄ κ°μ¬νκ² μ΅λλ€.
μ°¨λ¨μΌλ‘ νμν©λλ€.
μμμ΄μ.
μΆμ a4ce0eae43f7e0e2f91566ef2360830b86b9fdcf . @ShvetsKS νλ² λ³΄μ€λμ ?
a4ce0ea λ‘ μΆμ λ©λλ€. @ShvetsKS νλ² λ³΄μ€λμ ?
νμ νλ. νμ΄μ¬ μ¬μμ°κΈ°μμ λͺ¨λΈμ νλ ¨νλ λ° λμμ μ€ μ μμ΅λκΉ?
m2.load_model('xgb.model.bin') # load data
νλ ¨μ μ¬μ©λλ XGBoost λ²μ μ 무μμ΄λ©° μ νν μ΄λ€ λ§€κ°λ³μλ₯Ό μ 곡ν΄μΌ ν©λκΉ?
@ShvetsKS https://github.com/7starsea/xgboost-testing μμ λͺ¨λΈ νμΌ xgb.model.bin
μ μ»μ μ μμ΅λλ€ . λͺ¨λΈμ 1.0.0μΌλ‘ νλ ¨λμμ΅λλ€.
@ShvetsKS https://github.com/7starsea/xgboost-testing μμ λͺ¨λΈ νμΌ
xgb.model.bin
μ μ»μ μ μμ΅λλ€ . λͺ¨λΈμ 1.0.0μΌλ‘ νλ ¨λμμ΅λλ€.
λͺ¨λΈμ μ€μ λ‘ 1.2.1 λ° λ§€κ°λ³μλ‘ νλ ¨λμμ΅λλ€.
param = {'max_depth': 8, 'eta': 0.1, 'min_child_weight': 2, 'gamma': 1e-8, 'subsample': 0.6, 'nthread': 4}
κ°μ¬ ν΄μ.
μμ μ°¨μ΄λ λΆλ μμμ μ°μ°μ μμκ° λ³κ²½λμκΈ° λλ¬ΈμΈ κ² κ°μ΅λλ€.
_μ νν μ΄μ :_
a4ce0ea μ μ μ°λ¦¬λ λͺ¨λ λ무 μλ΅μ μ§μ λ³μ psum
(μ΄κΈ° 0κ³Ό λμΌ)λ‘ μ¦κ°μν€κ³ out_preds
μμ μ μ ν κ°μ μ¦κ°μν΅λλ€.
a4ce0eaμμ μ°λ¦¬λ κ° νΈλ¦¬ μλ΅μ μν΄ μ§μ out_preds
κ°μ μ¦κ°μν΅λλ€.
μμ μ΄ μ€λΉλμμ΅λλ€: https://github.com/dmlc/xgboost/pull/6384
@7starsea μ°¨μ΄μ μ μ°Ύμμ£Όμ μ κ°μ¬ν©λλ€. μμ μμ μ¬νμ νμΈν μ μμ΅λκΉ?
@hcho3 , @trivialfis κ·Έλ° μ°¨μ΄κ° λ―Έλμ μ€μνλ€κ³ μκ°νλμ? μΆλ‘ μ μν΄ λΆλ μμμ μ°μ°μ μμλ₯Ό λ³κ²½ν μ μλ€λ κ²μ΄ μ€μν μ νμΈ κ² κ°μ΅λλ€. κ·Έλ¬λ νλ ¨ λ¨κ³μλ λ΄κ° κΈ°μ΅νλ κ²κ³Ό κ°μ μꡬ μ¬νμ΄ μμ΅λλ€.
μ°λ¦¬λ κ·Έλ¬ν μ°¨μ΄λ₯Ό λ―Έλμ μ€μνλ€κ³ μκ°ν©λκΉ?
μΌλ°μ μΌλ‘ μμ΅λλ€. λ³κ²½ μ¬νμ μ΄ν΄λ³΄κ² μ΅λλ€. ;-)
@ShvetsKS λ°©κΈ νμΈν κ²°κ³Ό μ°¨μ΄λ μ΄μ μ νν 0μ λλ€. μμΈ‘ μ°¨μ΄λ₯Ό μμ ν΄ μ£Όμ μ κ°μ¬ν©λλ€.
@ShvetsKS
μ°λ¦¬λ κ·Έλ¬ν μ°¨μ΄λ₯Ό λ―Έλμ μ€μνλ€κ³ μκ°ν©λκΉ? μΆλ‘ μ μν΄ λΆλ μμμ μ°μ°μ μμλ₯Ό λ³κ²½ν μ μλλ‘ νλ μ€μν μ ν μ¬νμΈ κ² κ°μ΅λλ€.
μ€μ λ‘, μ°λ¦¬( @RAMitchell , @trivialfis λ° I)λ μ¬κΈ°μ λμν©λλ€. μμΈ‘μ μ νν μ¬νμ±μ μ무ννλ κ²μ λ³κ²½μ μννλ λ₯λ ₯μ μ¬κ°νκ² λ°©ν΄ν κ²μ λλ€. λΆλ μμμ μ°μ μ λΉμ°κ΄μΌλ‘ μ λͺ νλ―λ‘ μ«μ λͺ©λ‘μ ν©μ λ§μ μμμ λ°λΌ μ½κ°μ© λ€λ¦ λλ€.
XGBoost 1.2.0κ³Ό μ΅μ master
λΆκΈ° κ°μ μμΈ‘μ΄ μΌλ§λ λ³κ²½λλμ§ μ λννλ μ€νμ μ€ννμ΅λλ€.
1000κ°μ μλ‘ λ€λ₯Έ μμ μλλ‘ λ°μ΄ν°λ₯Ό μμ±ν λ€μ λ²μ 1.2.0κ³Ό λ§μ€ν°λ₯Ό λͺ¨λ μ¬μ©νμ¬ 1000κ°μ νλ ¬λ‘ μμΈ‘μ μ€ννμ΅λλ€. μμΈ‘μ λ³νλ μλ μ¬μ΄μμ μ½κ° λ°λμ§λ§ κ·Έ μ°¨μ΄λ κ²°μ½ 9.2e-7λ³΄λ€ ν¬μ§ μμΌλ―λ‘ μμΈ‘ λ³νλ λ Όλ¦¬ μ€λ₯κ° μλ λΆλ μμμ μ°μ λ‘ μΈν΄ λ°μνμ κ°λ₯μ±μ΄ ν½λλ€ .
μ€νμ© μ€ν¬λ¦½νΈ
**test.py**: λ€λ₯Έ μμμ μλλ‘ 1000κ°μ νλ ¬μ μμ±νκ³ μ΄μ λν μμΈ‘μ μ€νν©λλ€.
import numpy as np
import xgboost as xgb
import argparse
def main(args):
m2 = xgb.Booster({'nthread': '4'}) # init model
m2.load_model('xgb.model.bin') # load data
out = {}
for seed in range(1000):
rng = np.random.default_rng(seed=seed)
rx = rng.standard_normal(size=(100, 127 + 7 + 1))
rx = rx.astype(np.float32, order='C')
dtest = xgb.DMatrix(rx, missing=0.0)
out[str(seed)] = m2.predict(dtest)
np.savez(args.out_pred, **out)
if __name__ == '__main__':
parser = argparse.ArgumentParser()
parser.add_argument('--out-pred', type=str, required=True)
args = parser.parse_args()
main(args)
λͺ
λ Ή: `python test.py --out-pred [out.npz]`. Python νκ²½μ μ¬λ°λ₯Έ λ²μ μ XGBoostκ° μλμ§ νμΈνμμμ€. `xgb120.npz`κ° XGBoost 1.2.0μ λν κ²°κ³Όλ₯Ό μ μ₯νκ³ `xgblatest.npz`κ° μ΅μ λ§μ€ν°μ λν κ²°κ³Όλ₯Ό μ μ₯νλ€κ³ κ°μ ν©μλ€. **compare.py**: μμΈ‘ μ°¨μ΄μ λν νμ€ν κ·Έλ¨ νλ‘― λ§λ€κΈ°import numpy as np
import matplotlib.pyplot as plt
xgb120 = np.load('xgb120.npz')
xgblatest = np.load('xgblatest.npz')
percentile_pts = [50, 90, 99]
colors = ['tab:cyan', 'tab:olive', 'tab:green', 'tab:pink']
percentile = {}
for x in percentile_pts:
percentile[x] = []
percentile['max'] = []
for seed in range(1000):
diff = np.abs(xgb120[str(seed)] - xgblatest[str(seed)])
t = np.percentile(diff, percentile_pts)
for x, y in zip(percentile_pts, t):
percentile[x].append(y)
percentile['max'].append(np.max(diff))
bins = np.linspace(0, np.max(percentile['max']), 100)
idx = 0
for x in percentile_pts:
plt.hist(percentile[x], label=f'Percentile {x}%', bins=bins, alpha=0.8, color=colors[idx])
idx += 1
plt.hist(percentile['max'], label='Max', bins=bins, alpha=0.8, color=colors[idx])
plt.legend(loc='best')
plt.title('Distribution in prediction difference between XGBoost 1.2.0\nand master branch, tried over seed=[0..1000]')
plt.xlabel('Absolute difference')
plt.ylabel('Frequency')
plt.savefig('foobar.png', dpi=100)
μ¬κΈ°μ λ¬Έμ λ floatκ° μλ +
κ° κ·Έλ£Ήμ νμ±νμ§ μλλ€λ κ²μ΄λ―λ‘ ν©κ³λ₯Ό μ κ±°νμ¬ ν
μ€νΈν μ μμ΅λλ€. λ¨μΌ νΈλ¦¬μμ μμΈ‘ν©λλ€. κ²°κ³Όλ μ νν λμΌν΄μΌ ν©λλ€.
@trivialfis μ€μ λ‘ ntree_limit=1
μΈμλ₯Ό m2.predict()
μ μΆκ°νλ©΄ μ°¨μ΄κ° 0μΌλ‘ μ¬λΌμ§λλ€.
μμ²λ! κ·Έλμ λ€μμ κ·Έκ²μ λ¬Έμννλ λ°©λ² λλ λ¬Έμνν΄μΌ νλμ§ μ¬λΆμ λλ€.
λ΄κ° μκ² ν΄μ€. νμ¬λ‘μλ μ΄ λ¬Έμ κ° μ€μ λ‘ λ²κ·Έκ° μλλΌκ³ λ§νλ κ²μΌλ‘ μΆ©λΆν©λλ€.
κ°μ₯ μ μ©ν λκΈ
@ShvetsKS
μ€μ λ‘, μ°λ¦¬( @RAMitchell , @trivialfis λ° I)λ μ¬κΈ°μ λμν©λλ€. μμΈ‘μ μ νν μ¬νμ±μ μ무ννλ κ²μ λ³κ²½μ μννλ λ₯λ ₯μ μ¬κ°νκ² λ°©ν΄ν κ²μ λλ€. λΆλ μμμ μ°μ μ λΉμ°κ΄μΌλ‘ μ λͺ νλ―λ‘ μ«μ λͺ©λ‘μ ν©μ λ§μ μμμ λ°λΌ μ½κ°μ© λ€λ¦ λλ€.
XGBoost 1.2.0κ³Ό μ΅μ
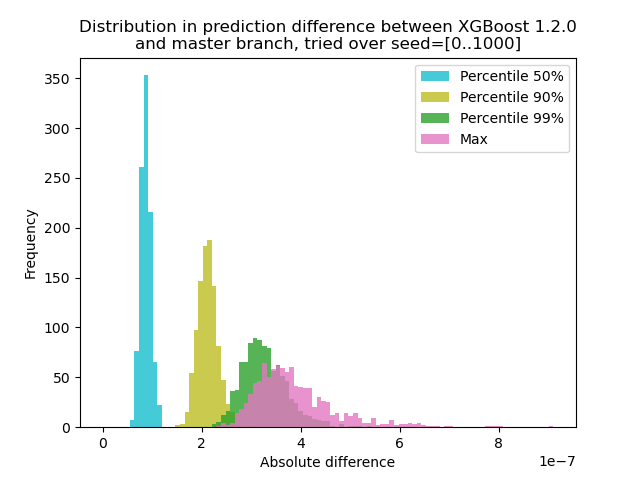
master
λΆκΈ° κ°μ μμΈ‘μ΄ μΌλ§λ λ³κ²½λλμ§ μ λννλ μ€νμ μ€ννμ΅λλ€.1000κ°μ μλ‘ λ€λ₯Έ μμ μλλ‘ λ°μ΄ν°λ₯Ό μμ±ν λ€μ λ²μ 1.2.0κ³Ό λ§μ€ν°λ₯Ό λͺ¨λ μ¬μ©νμ¬ 1000κ°μ νλ ¬λ‘ μμΈ‘μ μ€ννμ΅λλ€. μμΈ‘μ λ³νλ μλ μ¬μ΄μμ μ½κ° λ°λμ§λ§ κ·Έ μ°¨μ΄λ κ²°μ½ 9.2e-7λ³΄λ€ ν¬μ§ μμΌλ―λ‘ μμΈ‘ λ³νλ λ Όλ¦¬ μ€λ₯κ° μλ λΆλ μμμ μ°μ λ‘ μΈν΄ λ°μνμ κ°λ₯μ±μ΄ ν½λλ€ .
μ€νμ© μ€ν¬λ¦½νΈ
**test.py**: λ€λ₯Έ μμμ μλλ‘ 1000κ°μ νλ ¬μ μμ±νκ³ μ΄μ λν μμΈ‘μ μ€νν©λλ€.
λͺ λ Ή: `python test.py --out-pred [out.npz]`. Python νκ²½μ μ¬λ°λ₯Έ λ²μ μ XGBoostκ° μλμ§ νμΈνμμμ€. `xgb120.npz`κ° XGBoost 1.2.0μ λν κ²°κ³Όλ₯Ό μ μ₯νκ³ `xgblatest.npz`κ° μ΅μ λ§μ€ν°μ λν κ²°κ³Όλ₯Ό μ μ₯νλ€κ³ κ°μ ν©μλ€. **compare.py**: μμΈ‘ μ°¨μ΄μ λν νμ€ν κ·Έλ¨ νλ‘― λ§λ€κΈ°